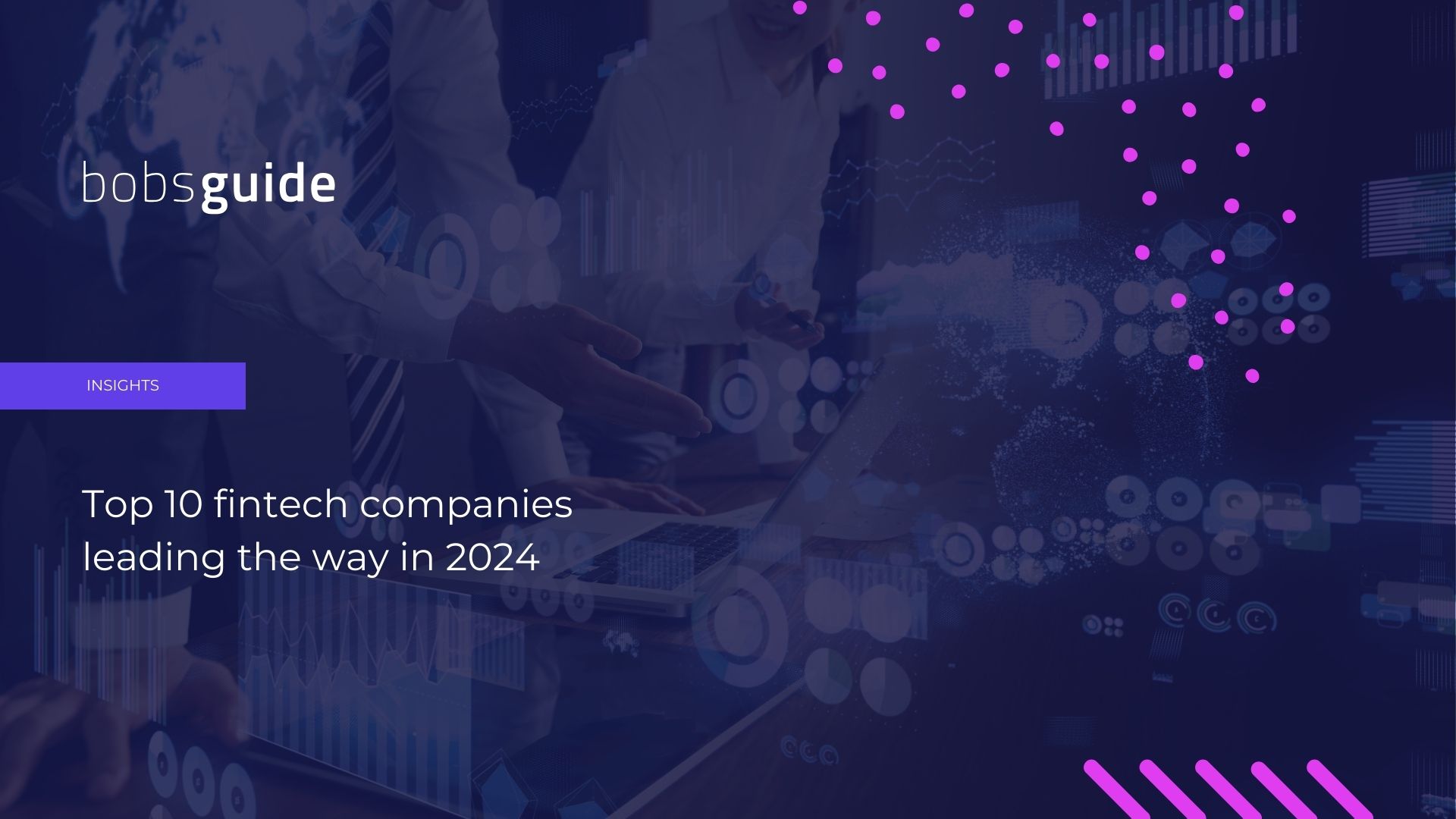
Fintech is the fusion of two of the largest and wealthiest sectors of the economy: finance and technology. As a result of this fusion, a highly valuable class of companies has emerged, leading innovation and the fintech industry. In this article, we present the top 10 fintech companies of 2024.
Financial technology, or fintech, is a dynamic sector reshaping the way companies and consumers conduct financial transactions. It is a fusion of finance and technology, where innovation is the driving force behind the creation of new tools and services that streamline and enhance financial activities.
The fintech market is burgeoning, with a projected value of $851 billion by 2030, growing at a compound annual growth rate (CAGR) of 18.5% from 2024 to 2030. This growth is fueled by the adoption of advanced technologies like artificial intelligence, blockchain, and application programming interfaces (APIs) that are revolutionising traditional financial services.
Fintech’s reach extends across various segments, including digital payments, lending, insurance, and wealth management, making it an indispensable part of the modern financial landscape Within this continuously flourishing industry, a class of top-tier companies has emerged as the frontiers of the fintech sector.
As of 2024, these are the ten largest privately held fintech companies worldwide that are expected to dominate the fintech sector in the years to come, according to Forbes Advisor:
Ant Group
Ant Group is the company behind Alipay, China’s largest digital payment platform. It is a testament to the global impact of fintech, having been founded in 2004 and headquartered in Hangzhou. Ant Group has revolutionsed financial transactions by providing digital wallet and payment app services to over one billion users worldwide. These services allow users to conduct transactions with a simple QR code scan, eliminating the need for physical cash or cards.
Despite facing regulatory challenges and a halted IPO in 2020, Ant Group’s valuation was recalibrated to $78.5 billion in 2023, highlighting its status as a fintech titan with a significant footprint in the digital economy.
Stripe, Inc.
Stripe, Inc., headquartered in both San Francisco and Dublin, Ireland, is a fintech company that has significantly simplified payment processing for businesses. Founded in 2009, Stripe has become a go-to platform for merchants seeking instant account approval and a seamless transaction experience.
In 2022, Stripe processed an impressive $817 billion in transactions, serving a diverse clientele that includes industry giants such as Amazon, Google, and Shopify. Despite a valuation decrease from $91 billion in 2021 to $50 billion in 2023, Stripe remains the most valuable fintech entity in the United States, showcasing resilience and adaptability in a fluctuating market.
Revolut
Revolut has emerged as Europe’s most valuable fintech company, with its headquarters in London, U.K. Since its inception in 2015, Revolut has redefined mobile banking, offering services that include international money transfers, a range of currency exchange options, and commission-free stock trading. Its app also supports cryptocurrency transactions, broadening its appeal.
With a valuation of $33 billion as of its last funding round in July 2021, Revolut has expanded its services globally, reaching markets beyond Europe, such as the United States and Japan. Despite concerns about its valuation in secondary markets, Revolut’s innovative financial solutions continue to disrupt the traditional banking sector.
Chime Financial, Inc.
Chime Financial, Inc., is a San Francisco-based fintech company that has established itself as a leading digital banking provider. Founded in 2012, Chime offers consumer-centric banking services that are technology-driven, designed to lower fees, and automate savings. Chime has a valuation of $25 billion, making it a compelling alternative to traditional banking institutions. Its primary focus is on providing a streamlined user experience and promoting customer financial health.
Chime’s innovative approach to banking includes features like early paycheck access and no overdraft fees, which have resonated with a growing customer base seeking more control over their finances.
Rapyd
Rapyd stands out as a fintech innovator specialising in global payment services. Founded in 2016 and headquartered in London, U.K., Rapyd has built a platform that facilitates cross-border payments through various methods, including bank transfers, cards, digital wallets, and cash. With a presence in over 190 countries, Rapyd aims to reduce the cost and complexity of financial transactions.
In early 2022, Rapyd’s valuation soared to $15 billion, a six-fold increase within a year, making it Israel’s most valuable private fintech company. Rapyd’s growth trajectory is marked by strategic acquisitions in Asia and Europe, enhancing its global payment network.
Plaid
Plaid operates as a critical intermediary in the fintech ecosystem, securely connecting financial accounts to apps and services. Launched in 2013 and based in San Francisco, Plaid’s technology enables consumers to link their bank accounts to financial applications like Betterment, Chime, and Venmo. It supports connections with 12,000 financial institutions, facilitating a seamless and secure data-sharing process.
Plaid’s last funding round in 2021 valued the company at $13.4 billion. Although it once considered merging with Visa, Plaid continues to thrive independently, leveraging investor capital to expand its operations and solidify its position as a linchpin of financial technology integration.
Brex, Inc.
Brex, Inc., headquartered in San Francisco, has made a name for itself in the fintech sector by offering financial services tailored for growing businesses. Founded in 2017, Brex provides an array of products, including credit cards, cash management accounts, and spend management tools, all designed to help companies scale efficiently.
With a valuation of $12.3 billion, Brex stands out for its innovative approach to business finance, combining seamless integration with robust financial controls. The company’s rapid growth and substantial valuation reflect its success in addressing the unique financial needs of emerging businesses in a competitive market landscape.
GoodLeap
GoodLeap, headquartered in Roseville, California, has established itself as a significant player in the sustainable home solutions market. Founded in 2003, GoodLeap provides a platform that facilitates financing for residential solar energy and other home efficiency solutions. With a valuation of $12 billion, GoodLeap is at the forefront of promoting eco-friendly investments, making it easier for homeowners to adopt sustainable technologies.
The company’s innovative financing model has not only contributed to the growth of clean energy but also reflects a broader trend in fintech companies supporting environmentally conscious consumer choices and contributing to a greener economy.
Bolt
Bolt, based in San Francisco and founded in 2014, has redefined e-commerce with its one-click checkout software solution. By streamlining the checkout process, Bolt has significantly reduced cart abandonment rates, enhancing the online shopping experience. Customers can effortlessly complete purchases with a single account across Bolt’s network. At its last funding round, Bolt achieved an $11 billion valuation, a testament to its innovative approach to e-commerce transactions.
However, recent challenges have led to workforce reductions, suggesting that Bolt’s actual market value might be lower than its peak valuation, reflecting the volatile nature of fintech valuations in a rapidly evolving industry.
Checkout.com
Checkout.com, a London-based fintech company, has carved out a significant niche in the online payment processing industry. Founded in 2012, Checkout.com has developed a robust platform that supports businesses with comprehensive, secure, and reliable online payment solutions. With a valuation of $11 billion, Checkout.com has distinguished itself through its ability to handle a wide array of payment methods, catering to a global clientele.
The company’s success is indicative of the fintech industry’s shift towards providing more integrated and user-friendly payment experiences, which are essential in today’s digital-first economy. Checkout.com’s growth trajectory underscores the increasing demand for fintech solutions that can adapt to diverse market needs.
全文來源:https://www.bobsguide.com/top-10-fintech-companies-leading-the-way-in-2024//